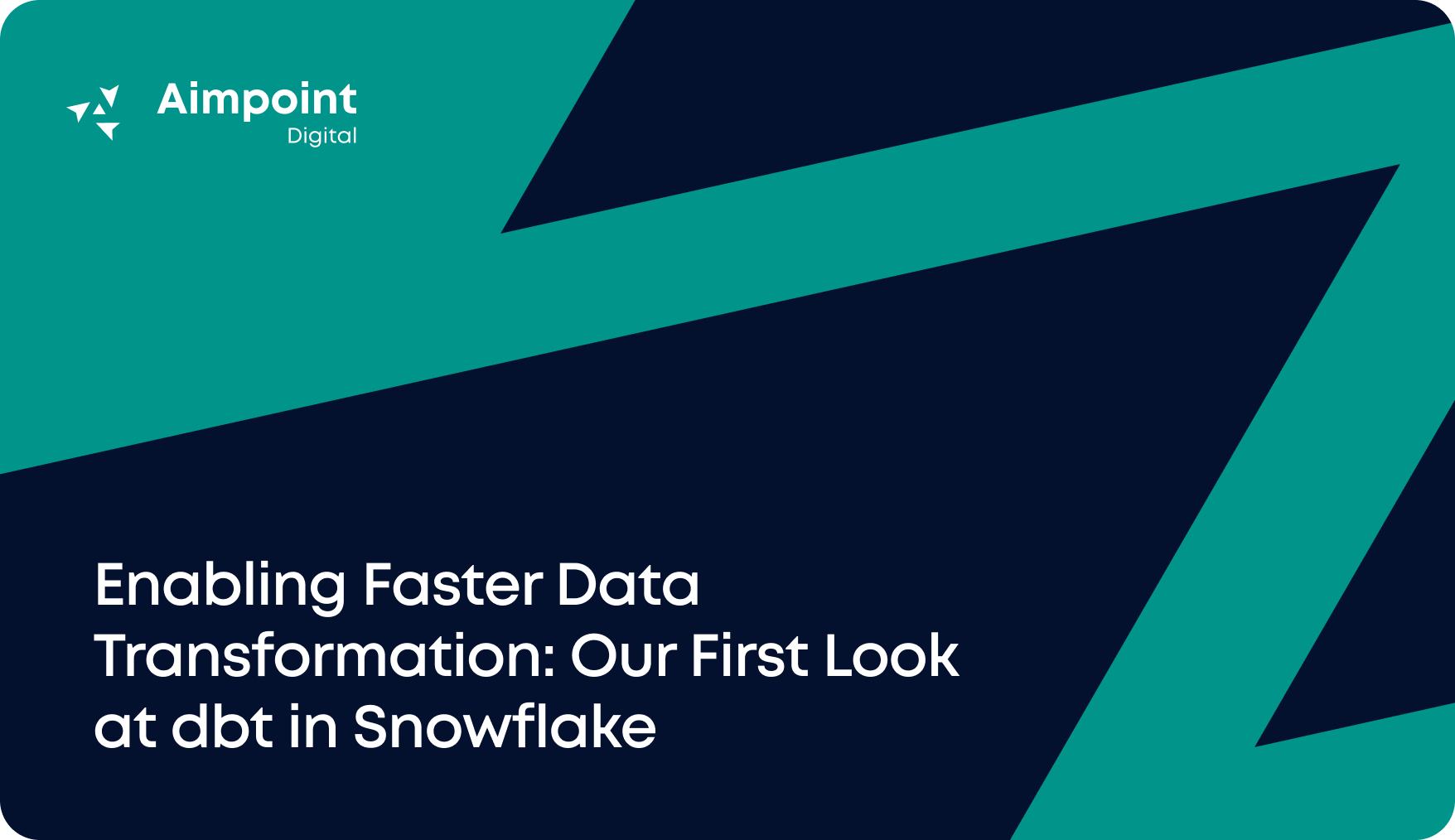
Data Engineering & Infrastructure
The ability to predict and prevent customer churn can be the difference between growth and decline for a business. Beyond direct revenue loss, churn significantly diminishes return on advertising investments through reduced lifetime value. Moreover, studies show that new customer acquisition can cost anywhere between five and seven times more than retaining a current one. Customer churn represents a critical challenge for Business to Business (B2B) Software as a Service (SaaS) enterprises.
Broad stroke approaches to retention are often cost ineffective and can even prompt churn in customers that would not have left. Additionally, in many cases the underlying data presents several inconsistencies that need to be addressed by synthesizing business knowledge with technical expertise which, if left unaddressed, leads to ineffective churn modeling. These combined effects make churn identification and management more challenging to execute than expected for SaaS companies.
To address these challenges, we used Databricks as a solution for both data management and model development. In collaboration with our Data Engineering & Infrastructure (DEI) team, we at Aimpoint Digital helped the client establish Databricks environments, access regularly updated data, and integrate CI/CD best practices using Databricks Asset Bundles. This setup provided a solid foundation to develop a robust and scalable ML model pipeline to predict customer churn-risk.
Our client, a leading B2B information technology provider faced rising churn rates without the tools to identify at-risk accounts or guide effective retention strategies. While churn prediction is inherently complex, B2B environments introduce unique challenges not typically seen in Business to Consumer (B2C) world. In this engagement, a key hurdle was the fragmentation of data across several teams. Prior to this project, teams were relatively siloed and there was no consolidated effort to bring all the data related to a customer together into one place. Additionally, customer behavior was found to be reactive—often triggered only by company outreach—resulting in minimal engagement prior to renewal cycles and limited visibility into account health.
Working closely with the client’s business and technology teams, we designed a solution architecture that laid out a plan to build an end-to-end ML pipeline to score customers based on their propensity to churn. These scores would then be used to formulate retention plans and prioritize efforts. The full solution was developed and deployed in the client’s Databricks environment over 24 weeks.
Before starting model development, our Data Engineering & Infrastructure team partnered with the client’s data warehousing team to build their Databricks environment from the ground up. Configuring multiple environments for development, staging, and production, the DE&I team implemented robust data pipelines to ingest information from a variety of source systems—all made easily accessible to users on Databricks’ Unity Catalog. With clean, up-to-date, and centralized data, the Decision Sciences team could focus entirely on building an effective churn model pipeline, confident in the reliability of the underlying data and infrastructure.
In addition to model development, partnering with our Decision Sciences team ensures you receive comprehensive support and expert advisory throughout the model integration process. From in-depth technical guidance across adjacent workstreams to clear, non-technical communication for executive alignment, the Aimpoint team is committed to driving successful adoption and implementation of our solutions. During this engagement, the Aimpoint team stepped into the role of technical manager, integrating directly into the client’s management tools to lead the team. We also onboarded and trained internal developers on Databricks, providing hands-on support as well as best practice recommendations.
Model development is just one piece of the puzzle—true impact comes from the collaboration, enablement, and insights that define a partnership with the Aimpoint team.
A strong churn model begins with a deep understanding of customer behaviors—how they engage with your business, why they leave, and what differentiates positive from negative experiences. Through collaboration with business leaders across multiple segmented teams, we consolidated insights from over 10 disparate teams. Working closely with data owners, we transformed this information into validated features, creating a unified, holistic view of each account’s life. Our model, taking in features quantifying account activity and account growth, produced a predicted churn risk score per customer.
To address limited behavioral data throughout much of the account lifecycle, we focused on key moments tied to renewal history. In addition to the time series features that represented changes in customer behavior over time, we also looked at specific points in an account’s life, identified through stakeholder collaboration, where changes in behaviors are expected. For instance, we learned anecdotally that the first three months of a contract are often the most critical for product adoption. Based on this insight, we developed logic that captured activity during the initial three months of every contract. This logic was then dynamically applied to all core behavioral features. This process of integrating business SMEs during feature engineering brainstorming allowed us to capture richer and more meaningful signals from an otherwise sparse behavioral dataset.
An often overlooked, but important step to experimentation is building a robust testing framework, which allows us to quickly evaluate incremental improvement from new features. By optimizing feature generation for Databricks compute, developing a custom model training and testing pipeline, and integrating automated tracking with MLflow, we reduced iterative runtimes from days to hours, allowing the team to understand the impact of newly developed features on the model that same day.
The churn model was deployed as part of a larger strategic initiative to improve retention. Through region-based test-control the solution helped contribute to a 2-percentage point reduction in churn and downsell. The delivered model and optimized pipelines enable ongoing refinement and seamless integration of new data, supporting continuous improvements in churn reduction beyond the engagement. With customer retention now a top organizational priority for the coming quarters, this model serves as a cornerstone of their retention strategy. Through our engagement, renewal representatives receive a churn risk score for each account they oversee, enabling them to focus their outreach efforts where they matter most.
Building on the momentum of this engagement, additional projects and workstreams—driven by our analysis and data—are already in motion to further support this critical initiative.
At Aimpoint Digital, we understand churn. We recognize that it is both a critical challenge and a uniquely complex issue for every organization. Our Decision Sciences team specializes in combining deep expertise of customer dynamics with the development of tailored strategies to align with your direct needs. If you or your organization is interested in learning more about how we can help support your retention goals, reach out to our team.
Whether you need advanced AI solutions, strategic data expertise, or tailored insights, our team is here to help.