Customer Behavior Modeling for Online Sportsbook
Learn how Aimpoint Digital leveraged Databricks' Machine Learning capabilities to assist a prominent betting and gaming company with the development of their proprietary Responsible Gaming Solution.
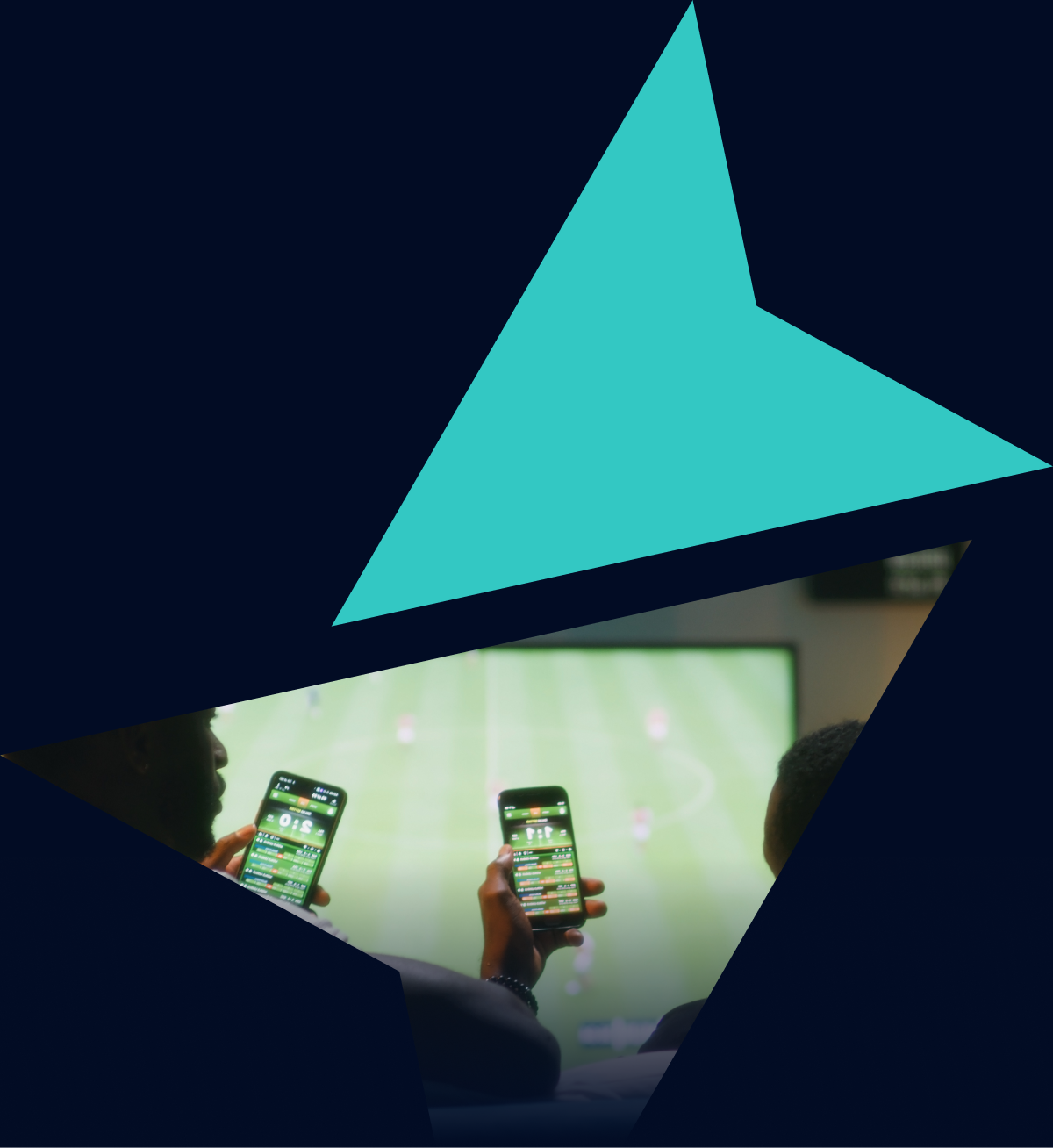
The Challenge
A nationwide betting and gaming company faced a significant challenge with their responsible gaming team, which was overwhelmed with manual reviews of users displaying potentially problematic behaviors. The primary indicator used was chasing losses—meaning they were continually increasing their stakes after losses. While this was a valid indicator in casino environments, it generated tens of thousands of false positives for sports betting users. Our client needed an efficient solution to accurately identify and prioritize problematic gamblers for manual review, reducing the burden on their responsible gaming team.
Our Approach
The Aimpoint Digital team developed an end-to-end machine learning (ML) solution within Databricks to address the client’s needs.
Our team started with an exhaustive research phase to gather as much context and insights from prior work in identifying problematic gambling behavior in similar industries.
The research insights guided the construction of a data pipeline to extract and engineer features from user behavior data. Then, an explainable propensity model was trained on user behavior and attributes to predict the likelihood of a user experiencing problematic gambling. An additional model was constructed to prioritize high-risk users based on the trend strength of their historical behavior. Finally, a comprehensive dashboard was built to report results and provide actionable insights.
To enhance the model’s effectiveness, the responsible gaming team was empowered to ground-truth label model predictions within the dashboard. This allowed the model to be regularly re-trained with these ground-truth labels, which continually improved its precision.
Results
The number of false positives sent to the responsible gaming team was reduced by thousands each day, allowing the team to focus on genuinely high-risk users. More time was focused on taking appropriate action for problematic users, rather than trying to identify them.
.png)
The dashboard became an integral part of the responsible gaming team’s daily routine, offering a centralized location to review historical user behavior and model predictions, access detailed explanations, and provide feedback to the model.
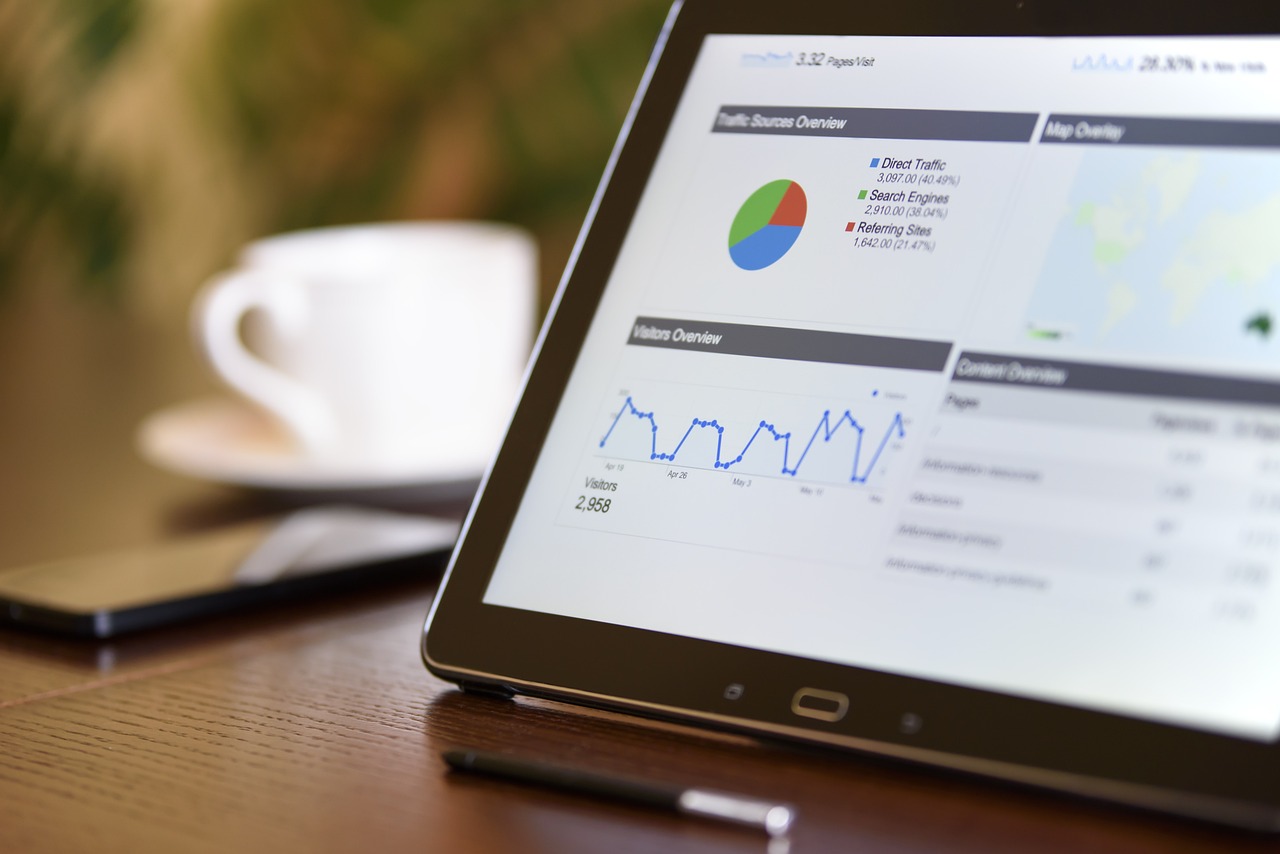
This streamlined approach not only enhanced the efficiency of the responsible gaming team but also ensured that high-risk users were promptly identified and addressed, supporting the company’s commitment to responsible gaming. The new dashboard also improved automation, giving our client the ability to review previous actions and sleep users for several days to prevent duplicate reviews.
.jpg)
Key Takeaways
The ML solution and dashboard significantly improved the client’s ability to manage and prioritize the review of high-risk customers. By reducing the false positives and integrating user-friendly tools, the responsible gaming team could operate more efficiently and effectively, ultimately leading to better outcomes for both the company and its customers.
Related case studies
Let’s talk data.
We’ll bring the solutions.
Whether you need advanced AI solutions, strategic data expertise, or tailored insights, our team is here to help.